Resources to learn more about AI Governance.
Get up-to-date details about the ideas, discussions, and processes around AI Governance, ML Observability and ML Ops.
Latest Articles
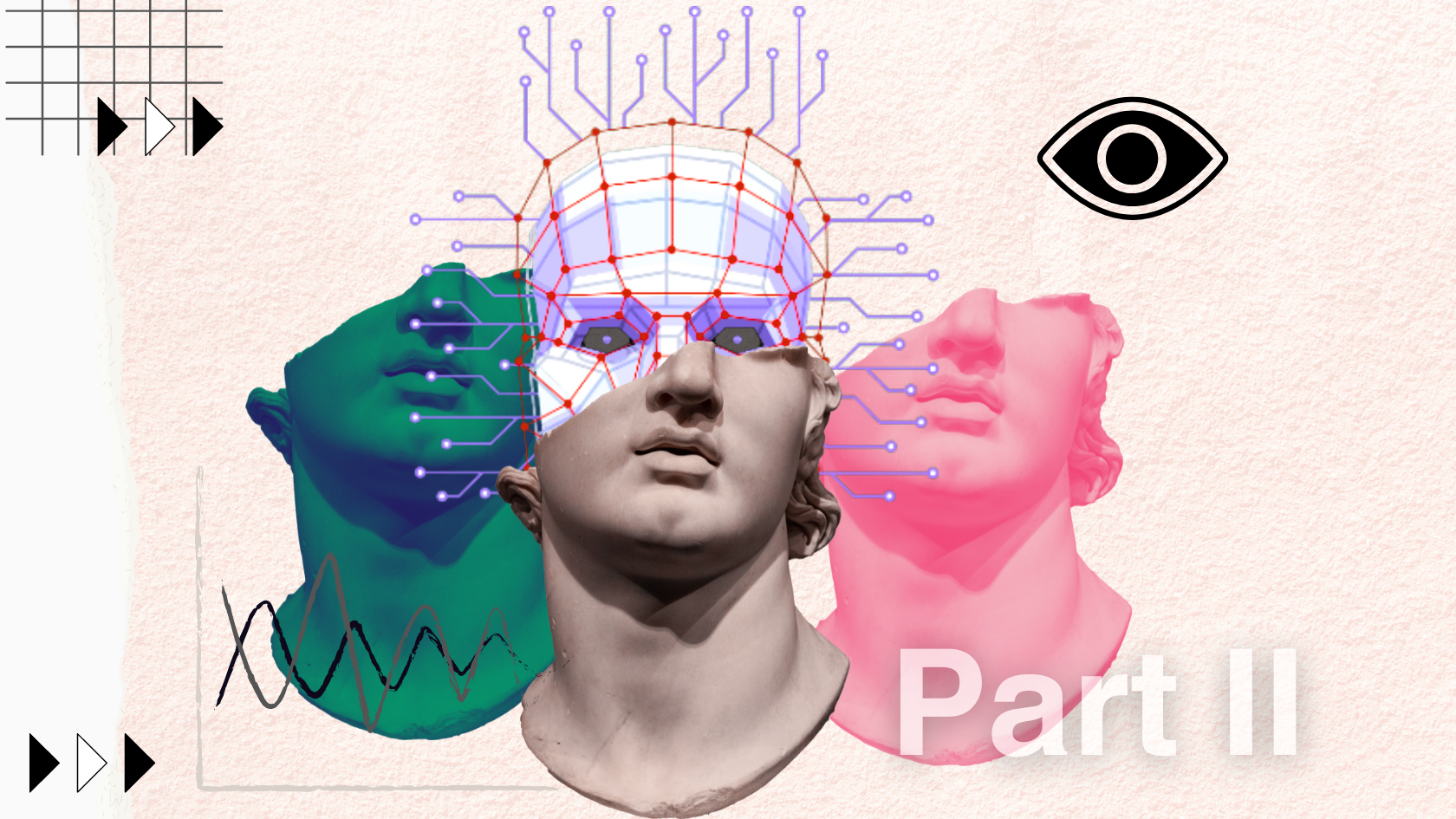
Privacy Preservation in the Age of Synthetic Data - Part II
Anonymeter, details of Anonymity Tests Using AryaXAI, and case study analysis
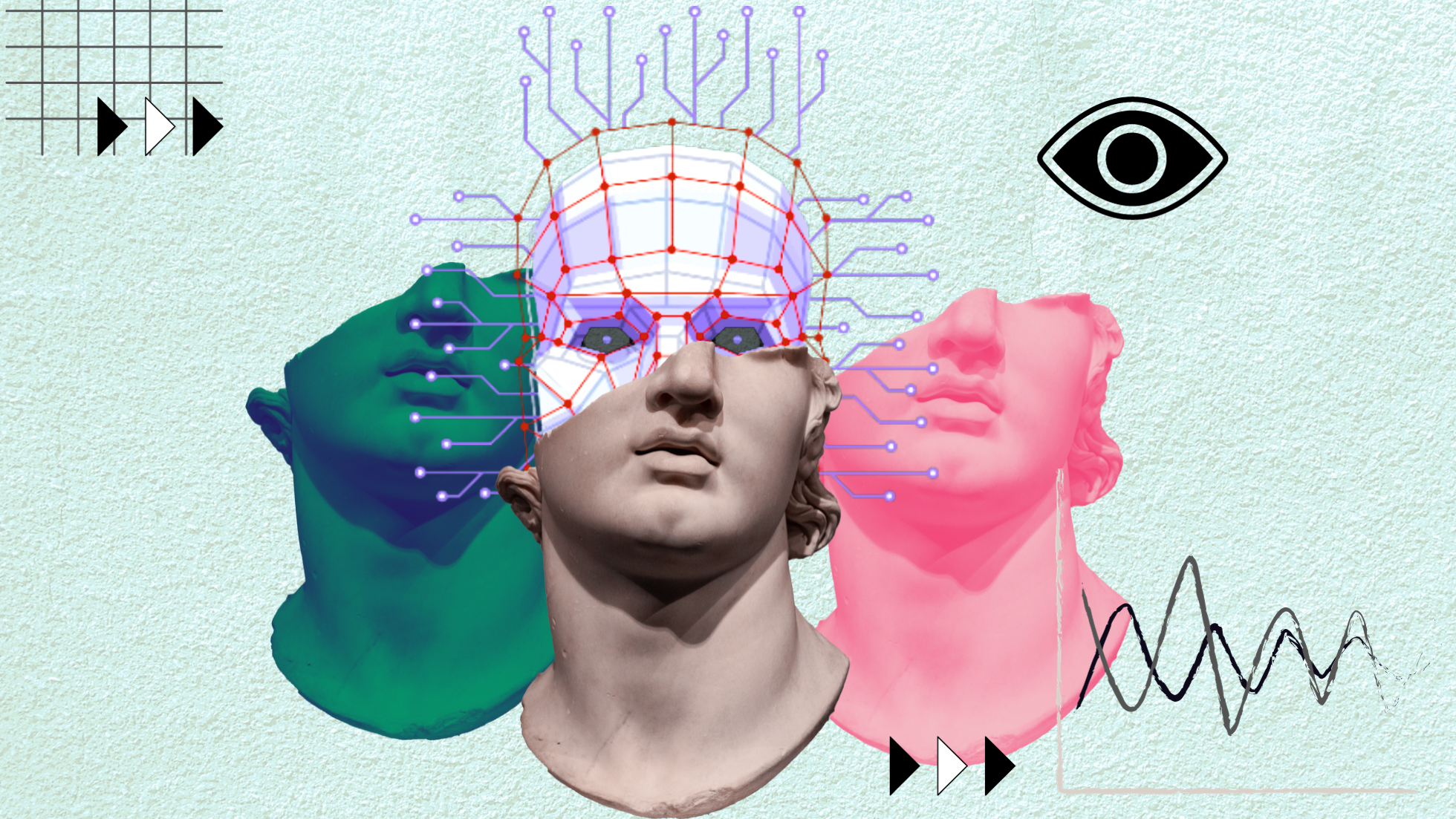
Privacy Preservation in the Age of Synthetic Data - Part I
Necessity of privacy risk metrics on synthetic data post-generation
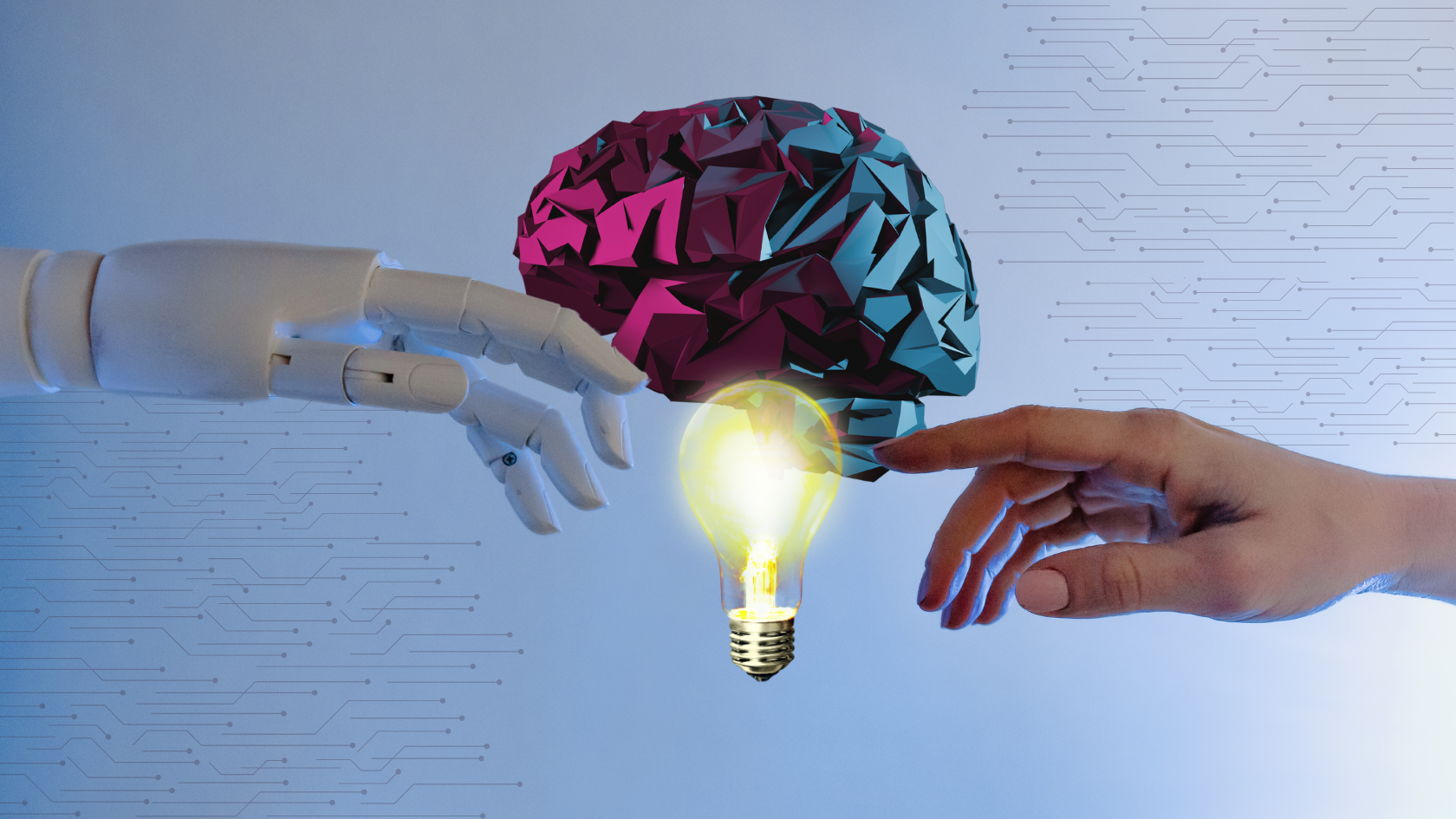
AI Broadcast Series #3: IP & copyright challenges for ‘AI’ solutions and the future of ‘AI’ regulations
Intellectual property (IP) complexities faced by next-gen AI applications
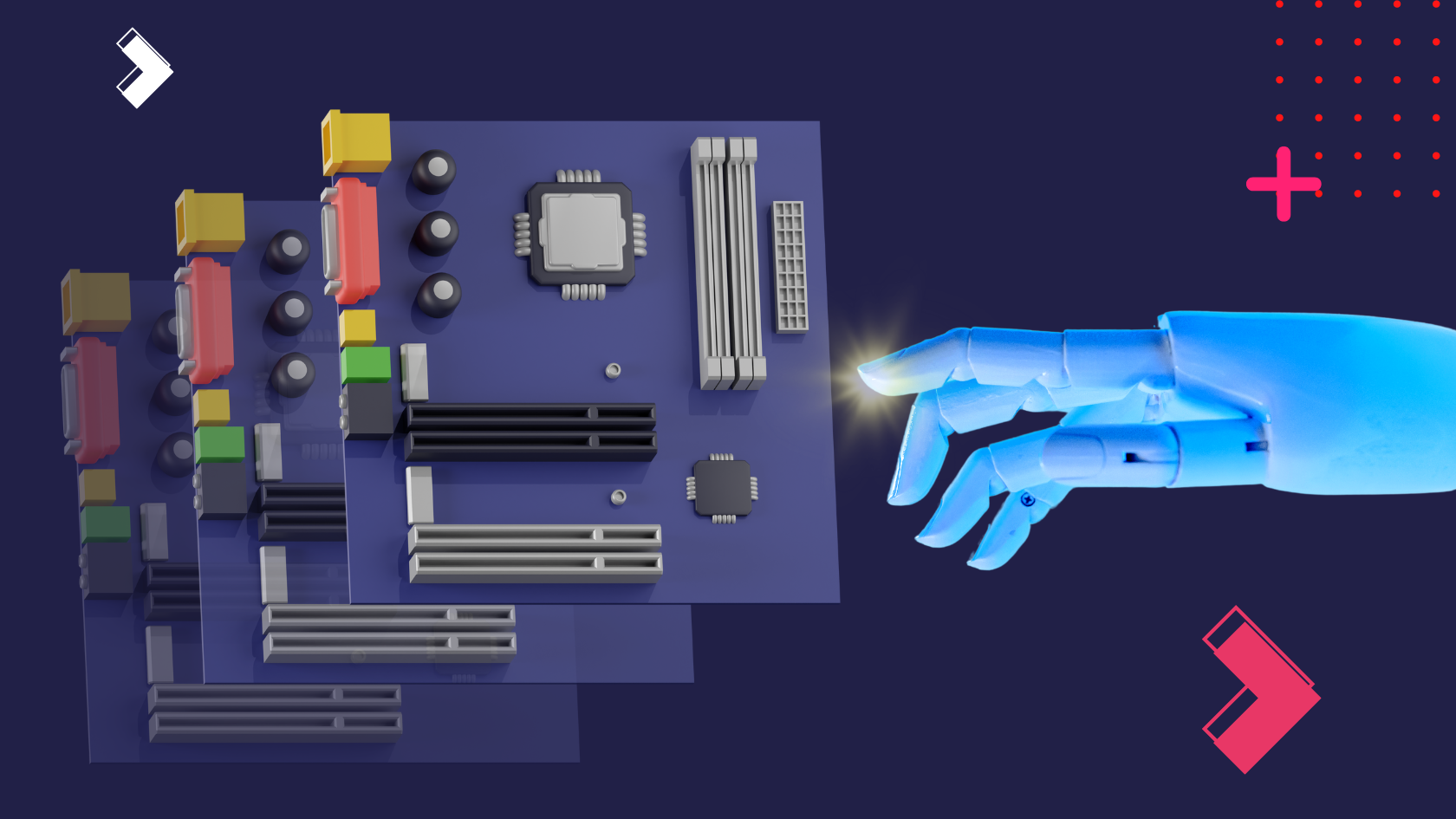
AI Broadcast Series #2: Why so much hype around AI Hardware? Going beyond Nvidia
Key factors influencing the growth trend in AI hardware, the growth of GPUs, companies and products in the market and the future of AI hardware
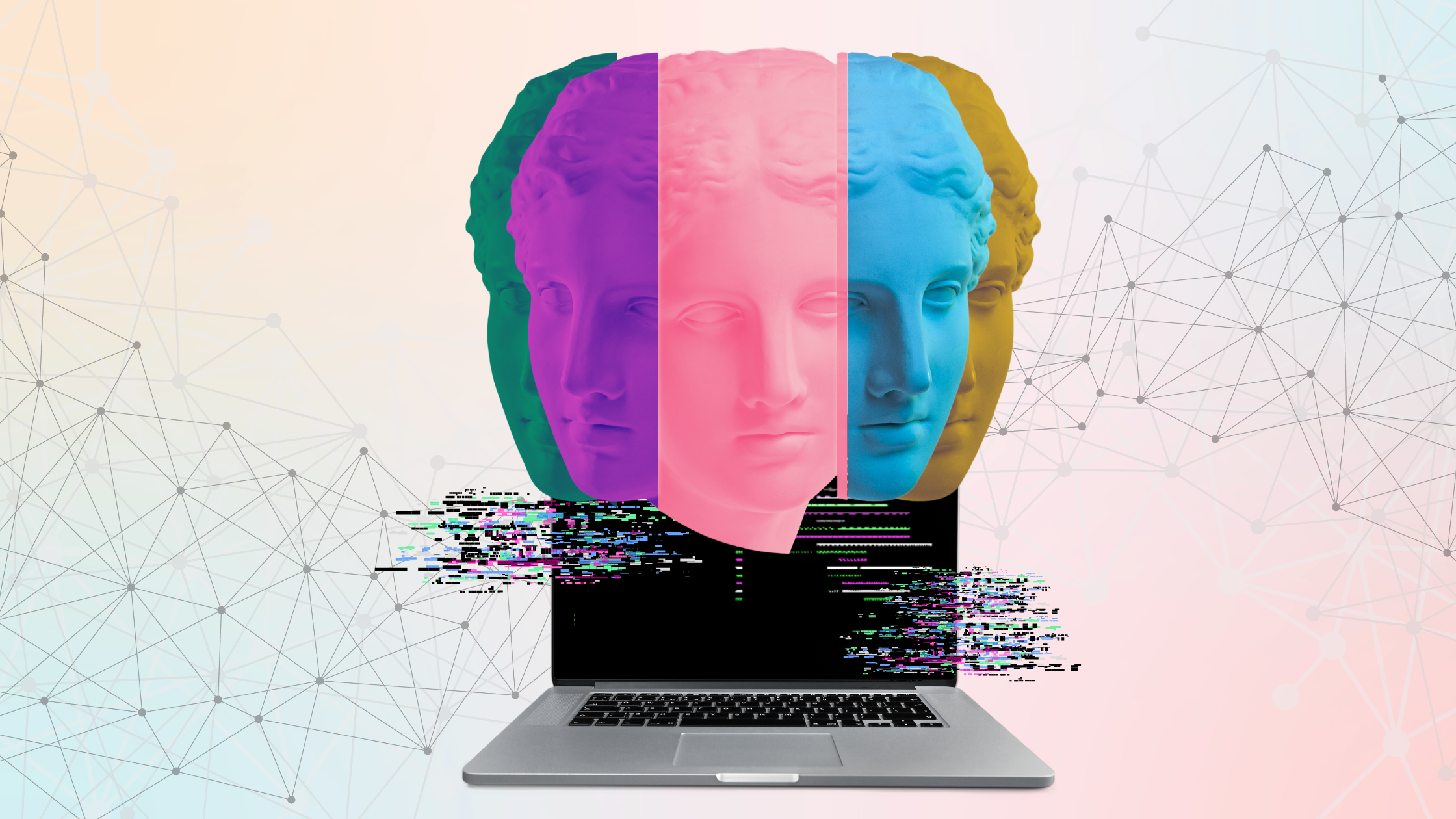
AryaXAI Synthetics: Delivering the promise of ML observability
Unlock a more effective approach to ML Observability
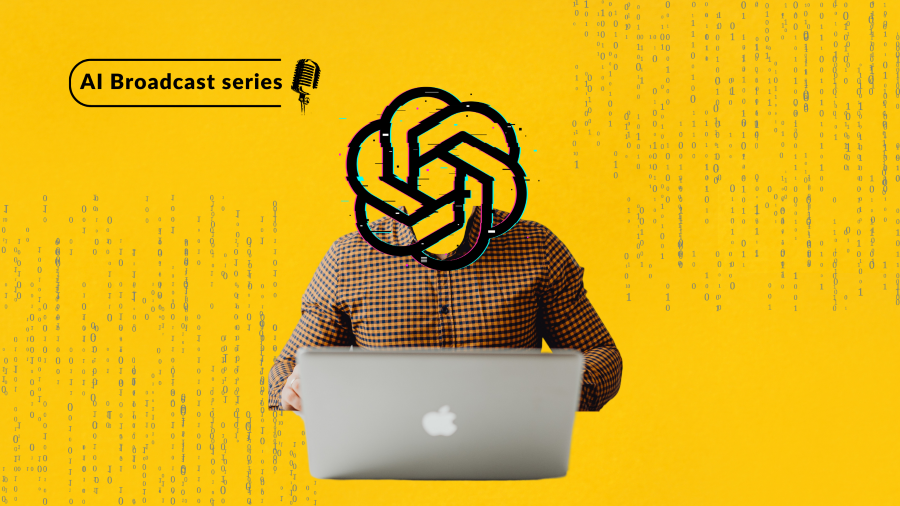
AI Broadcast Series #1 - What's next after ChatGPT in Banks, Insurers and financial services?
Delve into the world of ChatGPT and Generative AI, examine how the narrative around generative AI evolves and how businesses can stay ahead by adapting to the current advancements and anticipating future developments.
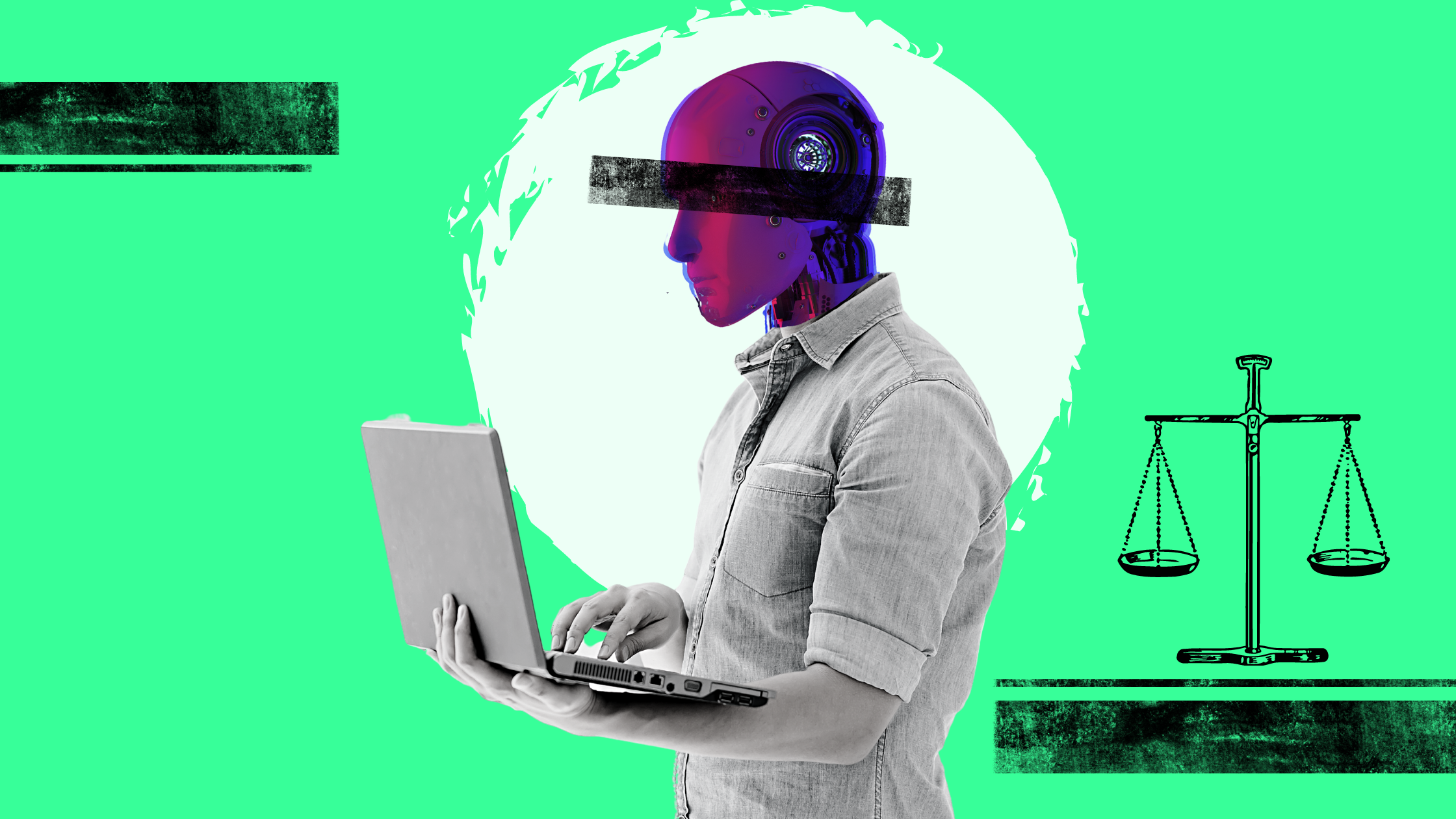
Navigating AI Bias: Global regulations and the quest for fairness
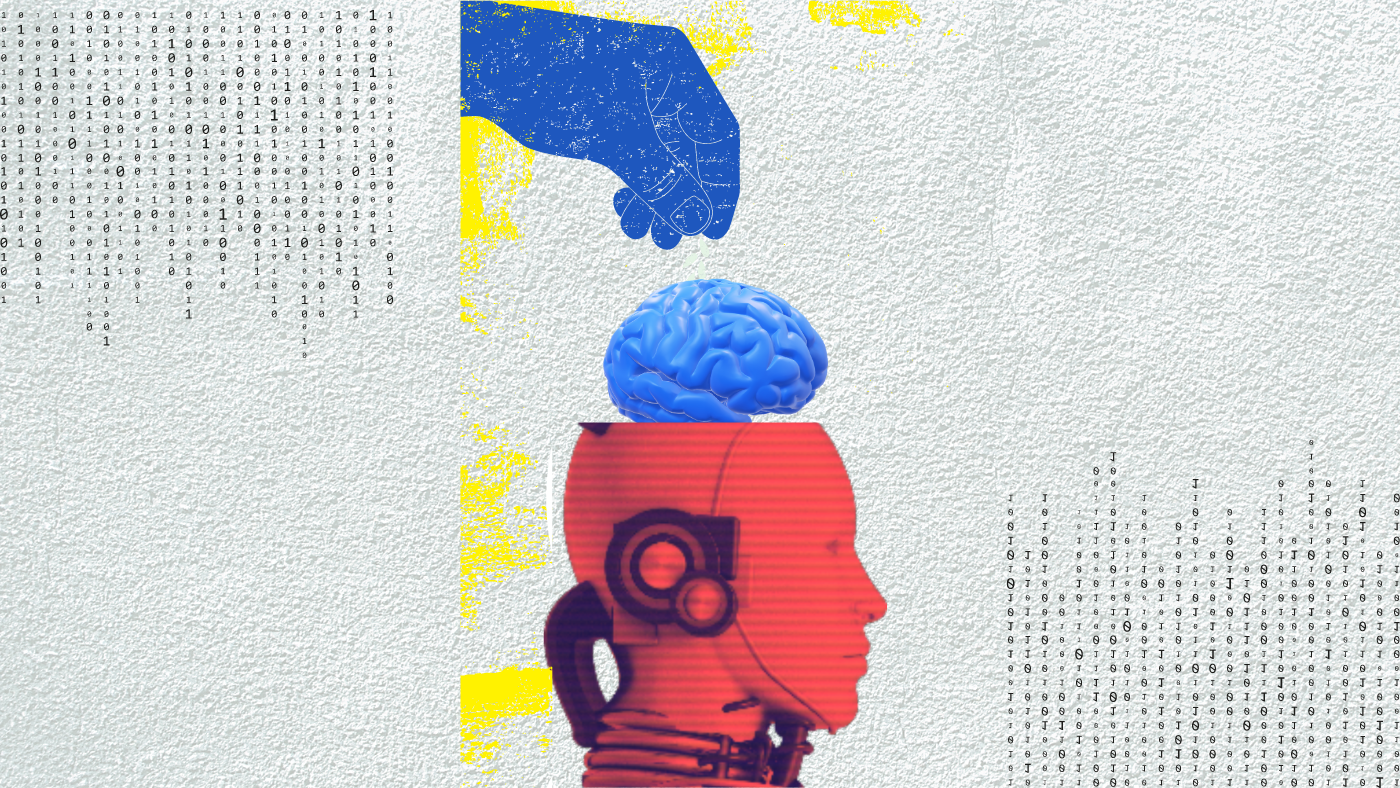
How to build Copilot using GPT4
Building vertical-specific copilots with GPT-4
Upcoming events
Latest Resources
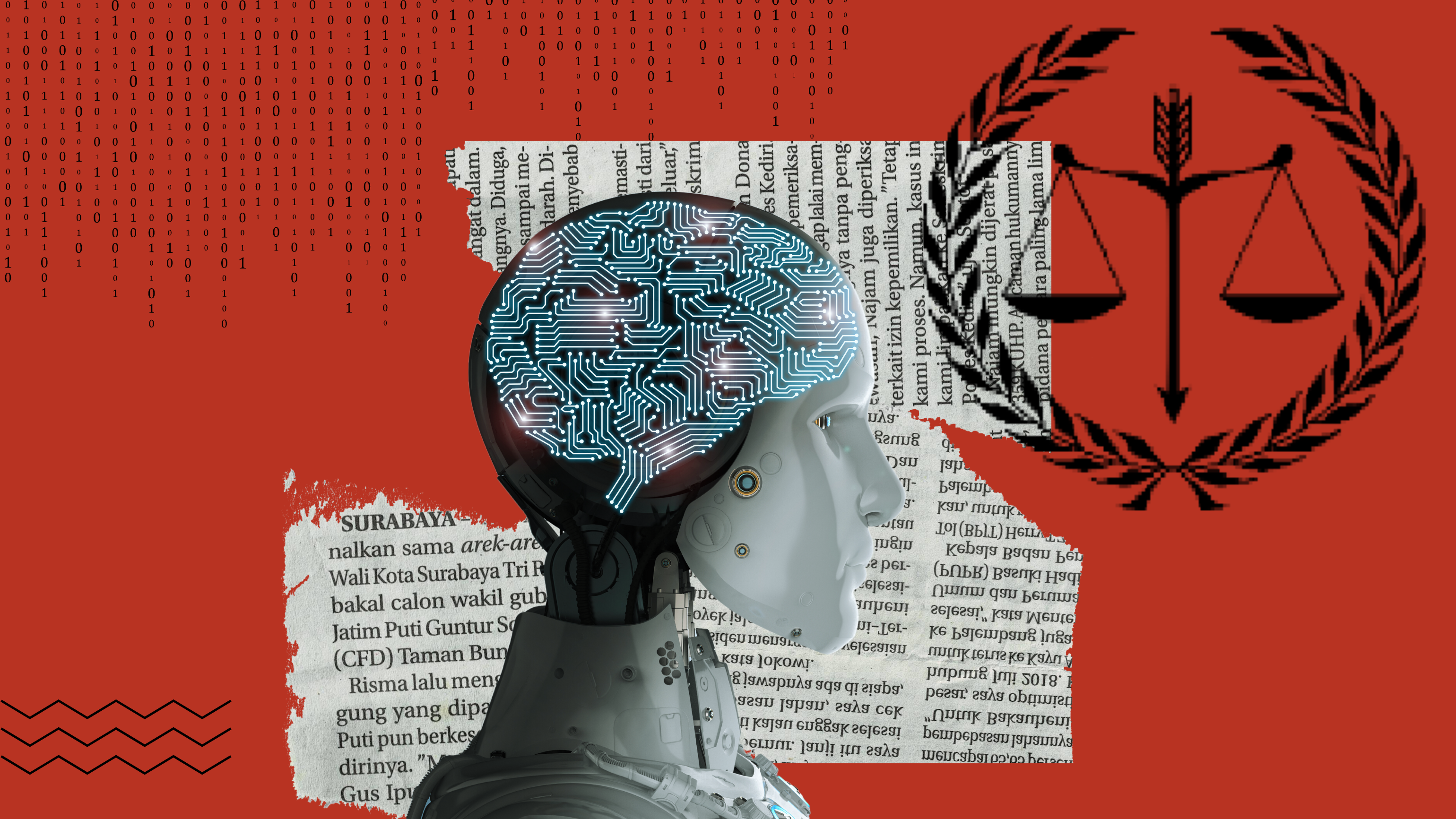
Whitepaper: The Evolving Landscape of AI Regulations in the US: Challenges, best practices and implementing effective AI Governance strategies
Emerging regulatory framework for AI in the US
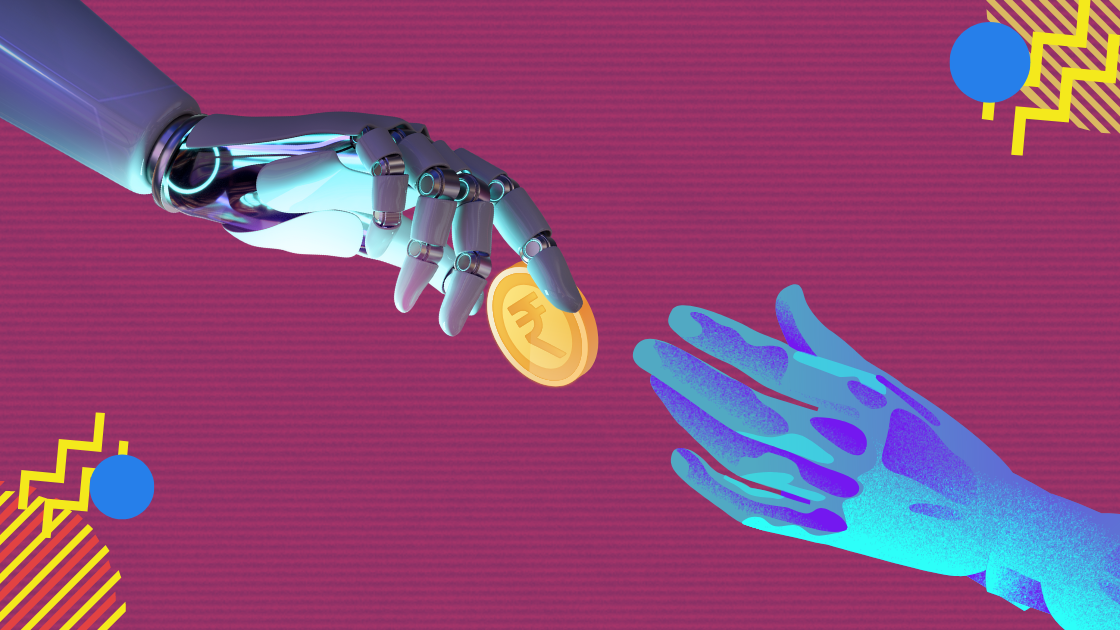
Whitepaper: AI Governance for Lending in India: Components & Challenges
Impact of RBI guidelines and components required for AI Governance

Policies and regulations around AI usage: Interpretation and impact
Overview of global Policies and regulations around AI usage, their sufficiency and impact on our future
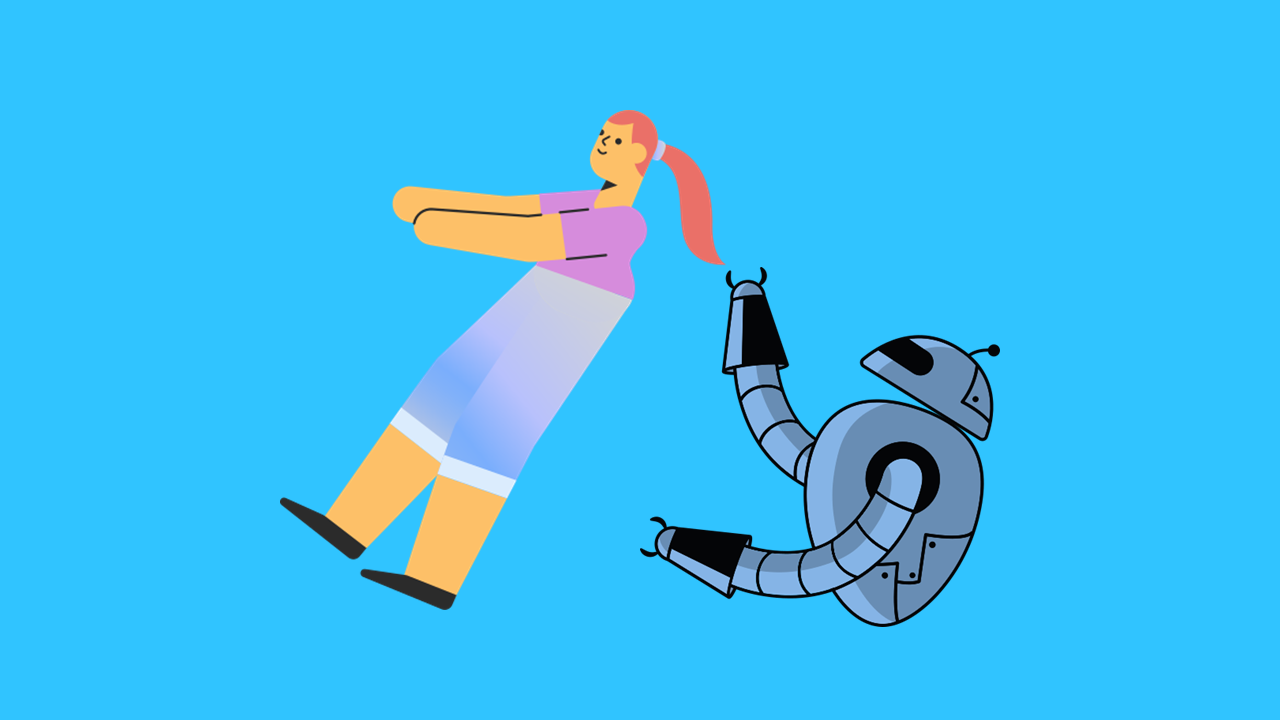
AI Explainability Framework in Financial Services: The Trust Imperative
Explore the current AI adoption in financial services, the ‘black box’ problem with AI and how explainability helps resolve the trade-off between accuracy, automation and being compliant
MLOps Wiki
This MLOps wiki offers a collection of clear explanations of the various MLOps concepts, their significance, and how they are managed throughout the ML lifecycle.
See how AryaXAI improves
ML Observability
Learn how to bring transparency & suitability to your AI Solutions, Explore relevant use cases for your team, and Get pricing information for XAI products.